MUMIP Meeting 4
MUMIP Meeting 4
Wednesday 4 October 2023
7:30pm UK / 12:30pm Boulder
Attendees
Jeff Beck (NOAA)
Judith Berner (NCAR)
Lisa Bengtsson (NOAA / CIRES)
Hannah Christensen (University of Oxford)
Mike Ek (NCAR / DTC)
Edward Groot (University of Oxford)
Hugo Lambert (University of Exeter)
John Methven (University of Reading)
Mark Muetzelfeldt (University of Reading)
Kathryn Newmann (NOAA / DTC)
Kasturi Singh (Imperial College London, soon to be University of Exeter)
Xia Sun (NOAA / DTC)
Apologies
Martin Leutbecher
Romain Roehrig
Nils Wedi
Agenda
1. Welcome to new members
Edward Groot has recently started at University of Oxford on the Leverhulme Trust grant
Kasturi Singh will soon start at University of Exeter on the Leverhulme Trust grant
Jeff Beck (NOAA) has recently joined the DTC collaboration as a subject expert
2. Updates from participating groups (all)
- Kathryn Newman and Xia Sun (NOAA NCAR DTC)
DTC funded project now extended to span four years – funding stretched over extra year to align better with other groups. Currently partway through year three of four (June 2023-May 2024).
Over past year (Y2), worked on running longer SCM simulations and refining diagnostics, to be shared today
Over coming year (Y3), will work on applying SCM tools and diagnostics to coarse-grained UFS high-resolution dataset for comparison
Key outcomes of Y2: scm-automation workflow developed, CCPP SCM run over ICON dataset. 6-hr SCM runs, reinitialised every three-hours, to span the 30-days. Two versions of CCPP: GFS_v17_p8 (typically used in global climate model, 100km resolution) and RAP physics (typically used in limited area high-resolution model, 15km resolution), both including parametrised convection.
Results were shown of domain mean and domain standard deviation for temperature and humidity for each version of CCPP versus coarse grained ICON. See some systematic differences. (see slides)
Also considered PDFs of temperature and moisture tendencies for each run compared with ICON, where the dynamics (advective) tendencies were subtracted from ICON to leave the physics component. For GFS and RAP, these could be split into contributions from different parametrisations (see slides)
Plan to produce high-resolution UFS simulation over Indian Ocean domain. Plan is to produce 6-hour runs, re-initialised (by downscaling GFS) every three hours, discarding the first three hours as spin-up. Reasoning being that global 3km is too expensive, necessitating limited area run, however long limited area runs will drift from the driving global GFS run.
(JM) Will there be issues with spin up with such a frequent reinitialization? Spin-up can be model dependent.
(HC) For ICON 1.5km 40-day simulation, recommended to discard the first 10 days. For CASCADE 4km 10-day limited area simulation, recommended to discard the first 24-hours
(XS) For this model, 12 hours may be sufficient
(KS) For WRF, 12 hours is typically discarded as spin up.
(LB) We could try the "Replay" methodology, which is a bit like nudging but dynamic. You use Incremental Analysis Update to nudge the large state to a pre-computed analysis (like ERA5)
(XS) Have updated CG methodology to work with UFS data. Issue with remapcon as don’t have cell corners. Can rempadis be used instead?
(HC) remapcon gives a “top hat” type averaging. Suspect that the grid corners be generated for a lat lon grid using cdo (HC to follow up)
Discussion opened on coordinating diagnostics for the model runs across the larger MUMIP group
(LB) Think about added value of this methodology over simply running different physics suites and high-res models? Key is intercomparison, and physics doesn’t feedback on dynamics – clean physics comparison
(HC) diagnostics shown today are climatological type diagnostics. But can also do a weather forecasting type diagnostics – compare for a given column what the CG ICON simulation said versus each SCM.
(LS) So given the same forcing, how does each SCM respond
(HC) Keen to start having a look at your data here in Oxford/Exeter
(LS) Value in multiple models – model independent information
(HC) Do you see multiplicative scaling in DTC runs to test SPPT?
(KN) Did look at conditional pdfs, but results were not as expected
(LS) Keen to start with repeating diagnostics from Christensen 2020 paper.
(JM) Conditional statistics – but domain not so large to sample e.g IOD, MJO
(HC) Diurnal variations. Ultimately like to have different areas as well
(XS) Interest in looking at weather patterns conditioned on different weather patterns
(EG) Interest in looking at diagnostics conditioned on convective systems with different levels of organisation. Lots of ideas of how to explore data
(JB) Interest in assessing SPP within the framework – links to Romain’s work. Also good to condition on percentiles as opposed to tendencies.
(LB) Use high-res ICON to say something about subgrid variability in state variables or e.g. TKE, to inform parametrisation schemes
(HC) Yes this can be done. Please indicate specific variables wanted to limit data volumes, and it will be computed.
- Hugo Lambert (University of Exeter)
Will start UM runs soon.
Recap on main interests at Exeter: summarising the behaviour of a range of parametrisations of the same process in terms of consistent known variables, to enable us to compare their behaviour with each other
E.g. Lambert et al, 2020 fitted a linear statistical model to convection in two parametrisations and a coarse-grained high-res dataset. Different behaviour in the two schemes and in observations, e.g. in how convection responds to a moistening BL (see slides)
Recent work has focused on clouds. Fit Gaussian Process emulator to model cloud amount as a function of large-scale variables for seven different models. Repeated in AMIP+4K simulations, and can see high level of robustness. (see slides)
Also have compared behaviour of evaporation in the tropics in a land surface perturbed parameter ensemble as a function of drivers to that in a range of AMIP models. Find PPE does not span the uncertainty space as represented by the AMIP models (see slides)
(JB) convection parametrised models need SPPT and SKEB, whereas convection permitting models need SPP for reliable forecasts. Spread is much bigger. Multi model ensembles represent uncertainty from bias as well as random error
(HL) Can see that parametrisations in different models are in different parts of the input space
(JB) want to get posterior of parameter distributions
3. AOB
(XS) Happy to share data. Data has been moved to Cheyenne/Campaign store. Contact JB for access.
(HL) JASMIN space? (HC to look into)
(XS) Going on leave November to February. Kathryn to be main contact in DTC
(JM) K-scale modelling meeting in the UK next week. Will attend and find out about more simulations available
ACTIONS
Agreed to use Teams in the next meeting
HC to follow up with XS re. coarse graining issues in cdo
JB to set up users with accounts on Cheyenne as needed for data access
HC to investigate Jasmin space
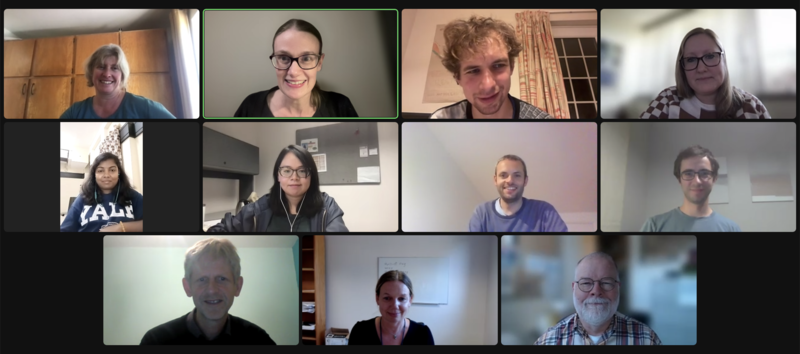
Attendees at MUMIP Meeting 4